

The graphical user interfaces (GUIs) are straightforward to use and its routines can be easily integrated within larger image analysis pipelines through the ImageJ macro language. NanoJ is also fully open-source and user-friendly.
#On1 photo raw 2018 gradiant map software#
NanoJ has been designed to integrate with the popular ImageJ or Fiji image analysis software and is easily installed as a standard set of plugins.
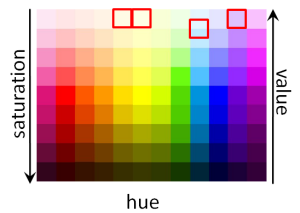
Thus, the NanoJ framework is capable of solving common imaging problems with broad biological applications and is compatible with a multitude of fluorescence microscope setups and experimental protocols. NanoJ (figure 1) is comprised of the following modules: NanoJ-Core-general image correction tools including drift correction and channel registration, both based on cross-correlation analysis NanoJ-SRRF-an analytical approach capable of extracting super-resolution data from a short sequence of diffraction-limited images, which can be acquired using most microscopes NanoJ-SQUIRREL-an algorithm to evaluate resolution and the presence of artefacts in super-resolution images NanoJ-VirusMapper-a single particle analysis method to generate nanoscale models of biological structures such as viruses NanoJ-Fluidics-a hardware and software framework to control fluidics devices, enabling automation of multiplexed experiments.
#On1 photo raw 2018 gradiant map series#
NanoJ is available as a series of ImageJ-based plugins which can be used independently or concomitantly. Here, we present NanoJ, a highly versatile set of image acquisition and analysis methods developed to improve the reliability and quantifiability of microscopy experiments, with a particular focus on the demands of live-cell SRM. Several SRM image processing packages are available, such as ThunderSTORM, LAMA and SIMcheck but each of these is focused on a specific type of SRM modality. Most SRM techniques use large quantities of raw data, often reaching several gigabytes to generate a single super-resolution image, thus requiring specialised high-performance image analysis tools.

In recent years, super-resolution microscopy (SRM) techniques have extended the spatial resolving power of fluorescence microscopy beyond the diffraction limit. Extraction of this biologically relevant quantitative information from fluorescence microscopy data typically requires digital image processing and analysis. It can reveal subcellular structures and interactions between specifically labelled molecules and allows the quantification of their dynamic behaviour in living cells. We expect to expand NanoJ in the future through the development of new tools designed to improve quantitative data analysis and measure the reliability of fluorescent microscopy studies.įluorescence microscopy has been ubiquitously used in biological studies since its invention in the 20th century. In this paper, we highlight the current capabilities of NanoJ for several essential processing steps: spatio-temporal alignment of raw data (NanoJ-Core), super-resolution image reconstruction (NanoJ-SRRF), image quality assessment (NanoJ-SQUIRREL), structural modelling (NanoJ-VirusMapper) and control of the sample environment (NanoJ-Fluidics). We named it NanoJ-a reference to the popular ImageJ software it was developed for. In recent years, our team has built an open-source image analysis framework for SRM designed to combine high performance and ease of use. This type of imaging often requires the use of specialised image analysis tools to process a large volume of recorded data and extract quantitative information. Super-resolution microscopy (SRM) has become essential for the study of nanoscale biological processes.
